Artificial Neurones Go Nanometric
A team of scientists has developed an artificial nanoneuron that mimics the behaviour of a brain neuron. Trained to recognise numbers spoken aloud, the component accomplished its task successfully. This cross-disciplinary work opens the way to a new architecture for the computers of the future.
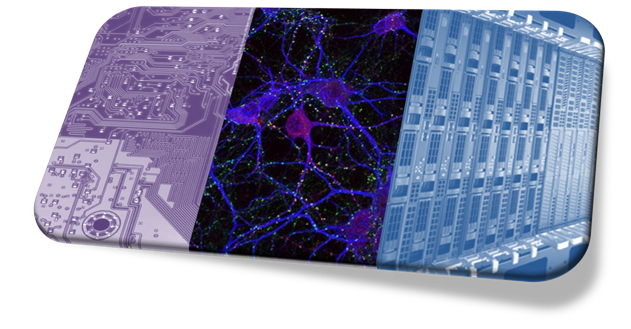
Photo © Prof. Dr. Julie Grollier
When will artificial intelligence (AI) exceed human performance? Posed by many scientists, industrialists and sci-fi enthusiasts, this question was the object of a study published in May 2017. The authors, scientists at the universities of Oxford in the United Kingdom, and Yale in the United States, explain how they questioned 350 experts on the future of their field. They thus established that there was a 50% chance that AI would exceed human intelligence in all tasks in forty-five years. These optimistic predictions echo recent phenomenal progress in one field in particular: deep learning.
Using this automatic learning technique, machines gradually unlock access to tasks that ordinarily only biological brains are able to achieve, such as recognising images or sounds. However, deep learning is not the only avenue being explored to enhance the abilities of our computers. More and more laboratories around the world are using a far less publicised yet promising path: developing artificial synapses and neurones. Rather than improving the algorithm, the goal is to develop a new architecture and use new materials to imitate the function of the biological brain as far as possible.
Working on the machine’s hardware rather than its software; this is the approach chosen by Julie Grollier, director of research at a joint CNRS-Thales unit. With her team last July, she published a study in the journal Nature that reports how the first nanometric-sized neurone (1 nanometre = 10-9 metre), or nanoneuron, was manufactured. The physicists explain that they were even able to test the capacities of their component by teaching it to recognise numbers spoken aloud - a task it completed successfully.
CALCULATION AND MEMORY
This work on the hardware architecture may seem obsolete compared to the sensational progress in deep learning. Just look at the example of the AI developed by DeepMind, a subsidiary of Google, to see how progress in this field is fast. While in January 2016, it beat a human champion playing Go (unexpected for at least another ten years), a new version of the AI called AlphaZero, beat its predecessor at the end of 2017 in just three days of training and without human help. The algorithm learned to become unbeatable at this centuries-old game by playing thousands of games against itself, with no instructions other than the rules and the position of the pieces on the board.
Despite this prowess, many scientists believe that deep learning alone is far from being a panacea. “This work was done using traditional von Neumann architecture, the same used for our personal computers”, recalls Alain Cappy from the University of Lille. While this is an ideal method of processing information for complex calculations such as digital simulations or data processing, it is not really suitable for various tasks such as image or sound recognition.” The problem lies in the physical separation between memory and calculation tasks in a computer.
In a traditional machine, the calculations are done by several billions of transistors, while the memory is on the hard disk. Some tasks require constant exchanges between calculation and memory (to make new calculations for example, you use previously calculated elements stored in the memory). The information has to move back and forth between the processing area and the memory area, wasting time and a large amount of energy. “Currently, when you use an image or sound recognition algorithm, the operation is too energy-intensive to be done on a personal device, which risks overheating”, explains Sylvain Saïghi, lecturer at Bordeaux University. “The data are sent to enormous servers that process the information before sending it back to the user.” This type of configuration has two major disadvantages: firstly, each operation consumes a lot of energy - the programmes that try to mimic the behaviour of neural networks consume about 10,000 times more power than the human brain. Secondly, this cannot be used for onboard systems that need real-time calculation, such as driverless cars.
Neuromorphic engineering appeared in this context. Its goal is to imitate the operation of the brain as well as possible using electronic components. Like many objects found in nature, our brain is a model in terms of calculating power, memory and consumption. In 2016, neuroscientists published a study in the journal e-Life, showing that our brain probably has a storage capacity 10 times larger than previously imagined. Thus it could contain 1 petaoctet (1015 octets), a capacity of similar magnitude to the entire worldwide web. In an adult, everything operates with about 20 watts of power, the equivalent of a low-energy light bulb. One of the secrets our brain hides to be so efficient was of particular interest to the neuromorphic scientists; unlike a traditional computer, calculation and memory operations are combined. Schematically, the neurons act as calculators and the synapses as memory. This architecture does not necessarily enable us to be calculation geniuses, but it does give us however a significant advantage when we need to recognise a cat or a mountain in an image. This structure, combining artificial neurons and synapses in the same circuit, is what the neuromorphic specialists are trying to recreate in the laboratory. “In the brain, the neurons are like oscillators, as they emit electrical pulses when they receive an input signal”, explains Julie Grollier. However, these oscillators do not behave linearly, in which simple excitation gives rise to a reaction. They actually accumulate the stimulations until they exceed a certain threshold, triggering the release of an electrical impulse. “This non-linearity of neurons is essential; this is what enables the brain to associate concepts”, confirms Sylvain Saïghi. “This is illustrated in the Pavlov experiment for example, which makes a dog salivate when it hears a bell, after stimulating it numerous times by presenting food simultaneously with the sound of the bell. The association happens naturally, as the neurons receiving the information are solicited at the same time.”
MAGNETIC MATERIALS
To develop a network of non-linear electronic oscillators and imitate the brain, some looked to existing technology. This is what Alain Cappy is doing, using CMOS (*) transistors from traditional machines, but differently to form networks of artificial neurons. “I believe that if we introduce new materials into this field, the devices will not get to the industrial stage before fifteen years, far too late”, argues the scientist. “The need is now!” Other scientists firmly believe that the solution lies in the development of new components, smaller and less energy-intensive than current technologies. “We think the human brain is comprised of 100 billion neurons, and even more synapses”, specifies Julie Grollier. “If we want to create an artificial brain one day containing a similar number of components, we will have to miniaturize them, otherwise we’ll have circuits measuring several metres long!” The research director and her team are developing the very first functional artificial nanoneuron. Rather than use a traditional semi-conductor, they looked into an original solution: spintronics. “When we try to reduce the size of transistors to the nanometre, we are quickly faced with major issues of stability over time”, explains Julie Grollier. Magnetic materials have the advantage of being very stable.”
The innovation of the scientists consists of a small cylinder, a few hundred nanometres in diameter, comprised of an insulating layer sandwiched between two magnets - two thin ferromagnetic plates, made of an alloy of iron and cobalt (see inset). The component operates on the spin of electrons circulating between two metal plates, a quantum property specific to each particle, that is often likened to kinetic moment. When a current is passed into the first ferromagnetic layer, the electrons take on a well-defined spin. Then, these electrons pass through the insulator using the tunnel effect, another quantum property that enables objects to cross a potential barrier if it is sufficiently thin. Once on the other side, the electrons transfer their spin to those in the second ferromagnetic layer. This causes the two magnets to oscillate like compasses. “The component has thus a short-term memory effect, linked to the brief relaxation time that follows the reception of the pulses”, explains Mathieu Riou, from the joint CNRS-Thales unit that participated in the study. This component reproduces quite faithfully the non-linear oscillation behaviour of biological neurons, which also have a form of short-term memory.
EASY TO MANUFACTURE
To test their innovation, the scientists used it to simulate a whole network of 400 neurons, with a strategy called time-division multiplexing. “The nanoneuron plays the role of each neuron in turn, a little like an actor who takes the role of 400 characters in a play”, decodes Mathieu Riou. Then the scientists recorded a large number of volunteers who spoke numbers aloud. The audio signals were converted into electrical signals and injected into the neuron to cause oscillation of the magnets. The transformation of the initial electrical wave into magnetic oscillation enables the neuron to make a calculation and gradually learn to recognise the numbers spoken.
“After training it for five minutes, our nanoneuron was able to identify the numbers with a 99.6% success rate”, rejoices Julie Grollier. “This is as good or even better than neurons that are 10,000 times larger!” Small, easy to manufacture, compatible with other technologies... Spintronic oscillators are very serious candidates for the future of neuromorphism. It just remains to assemble them in a network to try to develop a neuromorphic chip.
“It is an extremely interesting step for the field”, agrees Sylvain Saïghi, whose job is precisely to design neuromorphic systems comprised of several neurons. “Now we will be able to combine these nanoneurons with artificial nanometric synapses to create tiny complex systems!” While the specialists are saluting the elegance of this innovation, some like Alain Cappy, remain cautious about the future of these components: “For the moment, the learning process is not linked intrinsically to the component, but was done on a traditional computer”, notes the scientist. “Also, the nanoneuron remains quite energy-intensive, of the order of the microwatt. I believe that to have a valid network, we need to reduce consumption to a few picowatts (10-12 watts), as we can do using CMOS technology.”
Sylvain Saïghi shares these reservations about consumption but recalls that the energy spent is more important than the power: “These nanoneurons operate at an oscillation frequency of 1 billion hertz (gigahertz), which gives energy consumption of the order of the femtojoule, whereas CMOS technologies at best have a consumption of a hundred femtojoules. Not forgetting that no neuromorphic CMOS system is able to operate at such a speed.” Julie Grollier’s innovation has all the potential required to bring AI into the new era of spintronics. And who knows, if everything goes according to plan, may a few years from now, as well as their von Neumann architecture, machines will have artificial neurons and synapses at least as efficient as our own...
NANONEURON STRUCTURE
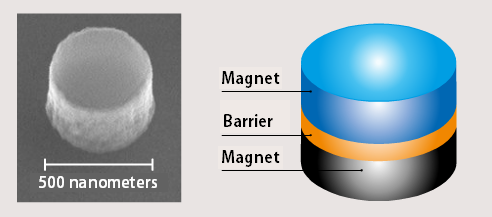
The nanoneuron of Julie Grollier’s team (left, seen under scanning microscope) is different in its structure (right): a thin insulting layer of magnesium oxide (yellow), sandwiched between an iron and boron magnet (blue) and another cobalt, iron and boron magnet (grey), used when an electrical current passes through it to reproduce the non-linear oscillation behaviour of biological neurons.
PATHWAY TO SENSORY SYSTEMS
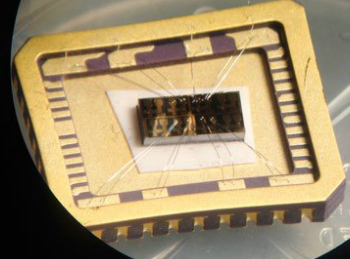
A few years ago, Julie Grollier, CNRS director of research, formed a second team to explore another avenue of neuromorphism. In a study published in journal Nature Communications in April 2018, the scientists explained they had developed a sensory nanoneuron, that imitates the behaviour of some populations of neurons in the brain. “We started from a hypothesis in which neurons specialising in interpreting sensory data have a stochastic behaviour, i.e. they react more randomly than deterministic neurons”, explains Damien Querlioz, CNRS researcher at Paris-Saclay university. This behaviour enables some neurons in our visual cortex for example, to identify the shapes or orientations of some objects less precisely, but more energy-efficiently. “To mimic this behaviour, we used the same magnetic component as before, but we designed it to be two to three times smaller”, adds Damien Querlioz. “Thus, the magnetic orientation of the component is less stable; it can change very easily.” Nine of these stochastic neurons were used to process some information, mainly mathematical operations. “This system is inspired by what happens in the part of the brain that receives impulses from the retina; the sensory neurons process the information to perceive shapes. We also made projections to estimate the behaviour of a system of 128 nanoneurons.” Using these components, the energy consumption is apparently 50 times less that an equivalent traditional electronic system. Once implemented, these components could be able to decipher or reproduce cursive handwriting.